Table of Contents
- Feeling Frustrated with A/B Testing?
- TL;DR: What you'll get from this A/B Testing Guide
- What Exactly is A/B Testing?
- Common Myths About A/B Testing
- Types of A/B Testing: AB, Multivariate, Split URLs and More
- Choosing the Right Shopify A/B Testing Platform
- Blend's Proven A/B Testing Process
- How Do You Prioritise A/B Tests
- Metrics to Track During A/B Tests
- When Should you NOT Run an A/B Test
- Real-World Shopify A/B Testing Wins
- What Happens when an A/B Test Doesn't Win
- What to Do When Your Test Doesn’t Deliver
- FAQs About Shopify A/B Testing
- Is A/B Testing Worth the Investment
Get useful eCommerce stuff
Improving conversion rates is vital for Shopify brands aiming to stay competitive. While there are many ways to achieve this, few approaches offer the reliability, clarity, and measurable outcomes that A/B testing does. At Blend Commerce, a specialist Shopify CRO agency, we've guided numerous brands to exceptional growth using strategic A/B testing.
In this guide, we'll explain the essentials of A/B testing, clarify common misconceptions, highlight successful examples from our clients, and answer frequently asked questions to help you effectively leverage this powerful tool.
Whether you're wondering where to start, which A/B testing platform to use, or how to measure success, this guide will provide the clarity you need.
Feeling Frustrated with A/B Testing?
If you've ever felt like your A/B tests just aren't delivering, you're not alone. Many brands come to us feeling stuck, unsure whether testing is even worth it. They’ve tried to run experiments, but keep hitting inconclusive results or struggling to know what to test next.
But here’s the thing: most failed A/B tests happen because they’re based on weak assumptions or trendy tactics, not real customer insight. That’s why we created our Free A/B Testing Kit, to give brands like yours a proven structure for planning, prioritising, and executing tests that drive real growth.
📥 Download the A/B Testing Kit
TL;DR: What you'll get from this A/B Testing Guide
- Clear explanation of A/B testing for Shopify
- Case studies showing real results (like +225% CVR)
- Platform comparisons to help you choose the right A/B testing tool
- A proven prioritisation framework (PECTI) to focus your testing
- Answers to the most common A/B testing questions
Jump to a Section
- What Exactly is A/B Testing
- Common Myths About A/B Testing
- Types of A/B Testing
- Choosing a Platform
- Blend's Testing Process
- Prioritisation with PECTI
- Case Studies
- FAQs
What Exactly is A/B Testing?
A/B testing compares two or more versions of a page or element to see which performs best. You test one change at a time to measure its impact on metrics like conversion rate, AOV, or revenue per visitor. It’s an essential component of effective Conversion Rate Optimisation (CRO).
Common Myths About A/B Testing
Myth: "A/B Testing is Dead"
Reality: It's not dead, it's just not done right.
Plenty of brands give up on A/B testing because they don't know how to do it properly. They ran weak tests with no data, declared it didn’t work, and moved on.
But here’s the truth: brands that test well, win big.
At Blend, we’ve used strategic, data-informed A/B testing to generate revenue lifts of 30%, 50%, or even 100% + for clients. By embracing testing as part of its growth engine, PerTronix saw a 65.16% increase in conversion rate and a 131.35% boost in revenue per visitor.
Dead? Hardly. Lazy testing is dead. Good testing is thriving.
Myth: "A/B Testing only provides Small Gains"
Reality: Small gains compound. And big ones still exist.
Yes, some tests only give you a 3 - 5% uplift. But stack ten of those together over 12 months, and you’ve got a completely different business.
And let’s not forget the big wins. Jackson’s saw a 225% increase in conversion rate from a single A/B test. Even "small" changes like moving a widget or adjusting copy can drive six-figure revenue shifts when you’ve got traffic.
The myth here isn’t that A/B testing only brings small gains. The myth is thinking you don’t need to compound them.
Myth: "A/B Testing is too Technical or Complicated"
Reality: It's only complicated if your process is broken.
Yes, testing can become a mess if you wing it, guess variations, skip QA, and don't align with your dev team.
That's why we created a bulletproof QAQC process. Every test at Blend goes through:
- Strategic hypothesis approval
- Wireframes and mockups
- Peer-reviewed Code Review and QA
- Final approval from our Head of Design & Development
- Monitored rollout and results analysis
Our clients (you) are involved at every key step, and nothing goes live without passing our checks. No guesswork. No chaos. No surprises.
A/B testing is only complicated if you're doing it wrong.
Myth: "A/B Testing Harms SEO"
Reality: Not if you follow the rules... and we do.
Google themselves have said it: A/B testing doesn't hurt SEO when implemented correctly.
Here's how we protect rankings:
- We use temporary 302 redirects, not 301s
- We apply canonical tags so Google knows what to index
- For on-page tests, search engines only see the default content
- We avoid testing during crawl-heavy site changes
If your SEO drops during a test, it's not the test, it's how it was set up. With the right implementation (ours), you're safe.
Types of A/B Testing: AB, Multivariate, Split URLs and More
-
AA Testing: Tests two identical variants to validate the testing setup, ensuring accuracy in data tracking, platform stability, and removing potential biases.
-
AB Testing: Compares a control (original) against a single new variant to assess improvements. At Blend, we use data-driven insights hypotheses, informed by detailed heuristic evaluations, analytics data, qualitative research, technical analysis, and competitor insights to measure impacts on key metrics such as conversion rate, average order value, and revenue per visitor.
-
ABC Testing: Compares the original against two different variants, allowing simultaneous testing of multiple hypotheses. For example, in our Jackson’s Homepage USP Optimisation test, three variants were tested simultaneously. Variant 1 saw a +225% increase in conversion rate and a +66% increase in revenue per visitor, significantly outperforming Variants 2 and 3. This demonstrates how ABC testing can provide deeper insights into customer behaviour and enable the selection of the most impactful solution.
-
Multivariate Testing (MVT): Multivariate Testing examines multiple elements on a single page at once to determine which combination delivers the best results. While A/B testing focuses on one change at a time, MVT analyses how changes to things like headlines, images, and buttons interact with each other.
It can uncover deeper insights but requires a much higher volume of traffic to reach statistical significance. Because of this, MVT isn’t usually the best starting point for most Shopify brands. At Blend, we typically recommend starting with A/B or ABC testing, especially if you're looking for clearer insights and faster wins.
-
Split URL Testing (Redirect Testing): Split URL Testing, also known as redirect testing, compares two or more completely different pages by sending traffic to different URLs. It’s ideal when you're testing bigger changes, like full redesigns, new page layouts, or changes to your navigation structure.
Because each version lives on a separate URL, this approach gives you more freedom to experiment with bold design or structural changes, without being limited to one page template.
Test Type | Best For | Recommended Traffic |
---|---|---|
AA Test | Testing two identical variants to validate setup and remove data tracking bias | 5,000+ monthly visits |
A/B Test | Single change (headline, CTA) | 10,000+ monthly visits |
ABC Test | Testing 2 variations at once | 30,000+ monthly visits |
Multivariate Test | Testing multiple elements at once | 50,000+ monthly visits |
Split URL Test | Layout or full-page redesigns | 15,000+ monthly visits |
Choosing the Right Shopify A/B Testing Platform
Your choice depends on specific business needs, and pricing generally depends on traffic volume. Some platforms charge based on the total number of visitors to your site, while others only charge for those included in A/B tests.
If you're unsure where to start, we discuss A/B testing strategy further in our CRO Audit Guide, which helps brands identify where to start testing based on data-driven insights.
Here's a breakdown of key platforms, along with their pros and cons:
Platform | Pros | Cons |
---|---|---|
Convert | Ideal for design-focused tests, flexible goal tracking, and integrations with GA4 & Clarity | Higher price point, lacks Shopify subscription metric support, moderate learning curve |
Omniconvert | Suited for personalisation, segmentation, and customer surveys | Steeper learning curve, setup can be heavy for eComm brands |
Intelligems | Excellent for pricing and free shipping tests, Shopify-integrated, strong on subscription metrics | More expensive than some competitors |
Shoplift | Native Shopify integration, easy setup, uses Shopify’s own metrics | Limited to A/B tests only, no custom goal setting |
VWO | Easy to use, strong segmentation, includes heatmaps and personalisation tools | Premium pricing for advanced features, dev support may be needed |
At Blend, we're tool-agnostic and work with whichever platform best suits your needs, ensuring smooth implementation and reliable insights.
Blend's Proven A/B Testing Process
At Blend Commerce, our A/B testing process is rooted in data and strategy, ensuring that every test is designed to deliver meaningful insights and measurable improvements. Our approach starts with a CRO Audit, which forms the foundation for all A/B testing efforts. Through this process, we identify key areas for testing and optimisation based on the following analyses:
-
Heuristic Analysis: Our strategists assess the user experience (UX) and identify barriers to conversion, considering navigation, layout, messaging, and trust factors.
-
Quantitative Analysis: We deep dive into analytics data from GA4, Shopify, and other tracking tools to uncover trends, friction points, and areas of opportunity.
-
Technical Analysis: Our developers assess page speed, site functionality, and technical health to ensure no underlying issues are negatively impacting performance.
-
Qualitative Analysis: We gather data from heatmaps, session recordings, customer surveys, and user journey tracking to understand how customers interact with your site.
- Competitor Benchmarking: We compare your store’s UX and performance against industry competitors, identifying potential strategic advantages.
From Insights to Action: How We Build and Execute A/B Tests
Once we have a comprehensive understanding of where opportunities exist, we move into hypothesis development and A/B test creation.
Our process includes:
-
Developing a Hypothesis: Based on data insights, we craft a hypothesis to test a specific UX or CRO improvement.
-
Wireframing & Design: Our team creates wireframes and Figma mockups to visualise the proposed changes.
-
Client Sign-Off: You review and approve the designs before development begins.
-
Development & QAQC: Our developers build the test variations in a controlled environment, following our rigorous QAQC process to ensure accuracy.
-
Test Deployment: We launch the test on your chosen A/B testing platform, gradually rolling it out to minimise risk.
-
Performance Monitoring: We continuously track key metrics (conversion rate, AOV, revenue per visitor, etc.), ensuring no negative impact on user experience.
-
Results & Insights: Once the test reaches statistical significance, we analyse the data, provide a report, and recommend the next steps.
By following this structured approach, we eliminate guesswork and ensure that every test contributes to measurable business growth.
How Do You Prioritise A/B Tests
Not every test will have the same impact on your revenue, and with limited resources, it’s crucial to prioritise the highest-value opportunities first. At Blend Commerce, we use our PECTI model to score and rank A/B testing hypotheses based on five key factors:
-
P – Proof: What evidence do we have that this test will work? (e.g., past test results, industry benchmarks, qualitative insights)
-
E – Ease: How complex is the implementation? Does it require extensive development work?
-
C – Cost: What is the financial investment required? This includes design, development, and third-party app costs.
-
T – Time: How long will it take to implement and see measurable results?
-
I – Impact: What is the potential uplift in conversion rate, AOV, or revenue per visitor?
Each test is scored on these criteria, generating a PECTI score out of 100 to help us objectively prioritise the most valuable optimisations.
How We Use PECTI to Maximise Results
-
High-impact tests come first
For example, checkout optimisations or product page enhancements tend to have the highest revenue potential, so we tackle these early. -
Lower-priority tests don’t get ignored
While small UI tweaks may not move the needle individually, they can compound over time, so we schedule them strategically. -
Reprioritisation is ongoing
We reassess test priorities each month in collaboration with our clients to ensure we’re always focusing on the areas with the greatest return.
Example: Applying PECTI in Action
In a recent project, we identified an opportunity to improve product information accessibility on a client’s Shopify store. Using our PECTI model, we prioritised a Tabbed vs Accordion layout test because:
-
We had strong Proof from heatmaps and user recordings showing visitors struggled to find key details.
-
The test was relatively Easy to implement, requiring only front-end development changes.
-
The Cost was low as it didn’t require third-party apps.
-
The expected Time to results was fast, users interact with product descriptions frequently, meaning we could achieve statistical significance quickly.
-
The projected Impact was high, as clearer product information could drive more confident purchase decisions.
The result? +11.17% increase in conversion rate and +18.73% uplift in revenue per visitor, confirming the effectiveness of our prioritisation model.
By using a structured scoring approach, we help Shopify brands focus on the A/B tests that deliver real, measurable business growth.
Metrics to Track During A/B Tests
Tracking the right metrics is crucial for evaluating the success of your A/B tests. At Blend Commerce, we focus on the following key performance indicators (KPIs) to measure the impact of optimisations:
- Conversion Rate: The percentage of visitors who complete a desired action, such as making a purchase. A successful A/B test should lead to a measurable uplift in conversions.
- Average Order Value (AOV): The average amount spent per transaction. If a test influences upsells, cross-sells, or product bundling, we track AOV to assess revenue impact.
- Revenue Per Visitor (RPV): A metric that combines conversion rate and AOV to determine the revenue impact of a test. This is particularly useful when evaluating checkout optimisations and pricing strategies.
- Add to Cart Rate: The percentage of users who add a product to their cart after viewing a product page. A high add-to-cart rate indicates strong purchase intent, making this a key engagement metric.
- Checkout Visits: The percentage of users who move from cart to checkout. If a test impacts checkout friction or trust signals, this metric will reveal its effectiveness.
- Bounce Rate: The percentage of visitors who leave without interacting further. A high bounce rate can indicate issues with page speed, messaging, or design layout, and successful tests often reduce bounce rates.
Each test is set up with a primary goal (e.g., increasing AOV, revenue per visitor or conversion rate), but we also track secondary metrics to assess additional impacts. Each test we run is designed to impact one or more of these KPIs, ensuring we drive meaningful and measurable growth for your Shopify store.
When Should you NOT Run an A/B Test
Not every moment is the right time to run a test. Here are a few red flags:
- During peak promotional periods: Campaigns like BFCM add too many variables.
- If your site has low traffic: You'll never reach statistical significance.
- Without a clear hypothesis: Don’t guess. Test what’s backed by data.
- When your tech setup isn't stable: Testing on a broken foundation will skew your results.
If you're unsure, start with a CRO Audit to find the best opportunities and get your site test-ready.
Real-World Shopify A/B Testing Wins
Pertronix: Moving Product Recommendation Widget Higher on PDP
Data Analysis & Hypothesis
Scroll map analysis revealed that the Applications section on product detail pages was preventing users from reaching the product recommendation module further down the page. This meant shoppers were missing opportunities to discover related products, especially problematic for users landing directly on a PDP from search or ads. However, removing the Applications section entirely wasn’t an option, as it was still a critical part of the decision-making process for many users.
We hypothesised that by moving the product recommendations higher and restructuring the Applications content, we could improve both discoverability and engagement, without sacrificing important product details.
Test Setup
- Variant 1: Moved the product recommendation widget above the Applications section.
- Variant 2: Same change as Variant 1, plus the Applications section was restructured into an accordion menu for cleaner presentation and easier access.
Test Results
Variant | Conversion Rate ↑ | Revenue Per Visitor ↑ | Add to Cart Rate ↑ |
---|---|---|---|
Original | – | – | – |
Variant 1 | +39% | +54.26% | +20.44% |
Variant 2 | +65.16% | +131.35% | +12.87% |
Insights & Recommendations
Both variants outperformed the original, but Variant 2 delivered the most significant lift, combining the benefit of improved visibility for recommendations with streamlined access to the Applications content via an accordion.
With results reaching 88–91% statistical significance, this test clearly validated our hypothesis. We recommended implementing Variant 2 permanently and using similar layouts in future PDP tests across other clients.
Titan Casket: Paradox of Choice and User Reviews on Collection Pages
Data Analysis & Hypothesis
Using behavioural analytics, we observed that users on collection pages were overwhelmed by the number of options, experiencing what’s commonly known as the paradox of choice. The absence of clear signals like user reviews or guidance markers made it difficult for visitors to confidently narrow down their selection.
Our hypothesis was that by reducing cognitive load and surfacing social proof (via star ratings), users would be more likely to engage with product listings, improve decision confidence, and complete their purchases faster.
Test Setup
- Variant 1: Introduced star ratings under each product tile on collection pages and made slight design tweaks to reduce visual noise, highlighting fewer but more meaningful choices.
Test Results
Device | Conversion Rate ↑ | Average Order Value ↑ | Revenue Per Visitor ↑ |
---|---|---|---|
All | +23.2% | +73.5% | +113.7% |
Mobile | +69.9% | +153.9% | +331.4% |
Desktop | +3.1% | +55.6% | +60.4% |
Insights & Recommendations
Despite not reaching the 95% statistical significance threshold (scoring 71.91% based on conversion count alone), the dramatic uplift in revenue, particularly on mobile, made the case for implementation clear.
By simplifying the decision-making process and building trust through visible user reviews, this test proved that often less can lead to more. We recommended permanently implementing this version and exploring further opportunities to reduce friction on high-traffic collection pages.
Jackson's: Above-the-Fold Optimisation
Data Analysis & Hypothesis
Scroll map data revealed that users were failing to see one of Jackson’s most compelling visual assets, its ingredient icons, due to their position lower on the homepage. These icons were key in building trust and interest, especially among health-conscious snackers.
We hypothesised that repositioning these icons higher on the page would increase visibility, drive engagement, and ultimately boost conversions. To test this, we created three variants:
- Variant 1: Moved ingredient icons above the fold and slightly altered the order and descriptions.
- Variant 2: Switched the placement of content but kept icons slightly lower.
- Variant 3: Displayed icons only, removing the supporting title and description.
Test Results:
Overall Performance
Variant | Conversion Rate ↑ | Revenue/User ↑ | Products/Visitor ↑ | PDP Visits ↑ | Add to Cart Clicks ↑ |
---|---|---|---|---|---|
Original | – | – | – | – | – |
Variant 1 | +225% | +66% | +88% | +2.19% | +56% |
Variant 2 | +127% | +58% | – | – | – |
Variant 3 | +44% | -3.8% | – | – | – |
Mobile Performance
Variant | Conversion Rate ↑ | Revenue/User ↑ |
---|---|---|
Variant 1 | +129% | +48% |
Variant 2 | +78% | -6% |
Variant 3 | +43% | -34% |
Desktop Performance
Variant | Conversion Rate ↑ | Revenue/User ↑ |
---|---|---|
Variant 1 | +89% | +109% |
Variant 2 | -8% | +251% |
Variant 3 | +59% | +75% |
Insights & Recommendations
Variant 1 consistently outperformed all other variants across every key metric on both mobile and desktop. The data confirmed that the repositioned ingredient icons and revised content hierarchy made the brand’s value proposition instantly clear to new and returning users.
While Variant 2 performed well for revenue on desktop, its negative impact on conversion rate and mixed performance on mobile made it less viable. Variant 3’s removal of supportive content reduced user clarity, leading to inconsistent and less favourable results.
Although the increased number of variants made statistical significance harder to achieve, the consistent upward trends observed throughout the test provided strong enough evidence to recommend a permanent implementation of Variant 1.
Want to see more examples of A/B tests in action? Explore our Micro Case Studies for detailed insights into the strategies, hypotheses, and outcomes behind our real client experiments.
What Happens when an A/B Test Doesn't Win
Not every A/B test will deliver a significant uplift in your key metrics, and that’s perfectly normal. In fact, around 1 in 3 A/B tests typically result in a “winning” variant, but that doesn’t mean the others are wasted effort.
At Blend Commerce, we view every test as a learning opportunity. A losing or inconclusive test can still provide valuable insights that sharpen your understanding of user behaviour and inform future experiments. For example:
- A variant that underperforms may indicate resistance to change in a certain area of the user journey.
- It may reveal that the problem wasn’t where we originally thought it was, redirecting attention to a more critical touchpoint.
- A test that doesn’t reach statistical significance can highlight insufficient traffic or seasonal behaviour, helping us better prioritise and time future tests.
- Most importantly, we learn what doesn’t work, which is just as important as learning what does.
When a test doesn’t perform as expected, we revisit and reprioritise it through our PECTI framework. This structured model helps us assess the evidence (Proof), ease of implementation, cost, time to impact, and potential uplift of each test idea. If a test needs to be refined, retimed, or replaced, we use PECTI to objectively evaluate its next best step, ensuring that your roadmap stays focused on the actions with the highest strategic value.
Ultimately, CRO isn’t about winning every test. It’s about continuous improvement, grounded in evidence, that drives long-term growth over time.
In short: unsuccessful tests aren't failures. They're part of a continuous optimisation cycle, helping to refine hypotheses and unlock bigger wins in future iterations.
What to Do When Your Test Doesn’t Deliver
If you’ve ever launched a test and felt unsure about what the result actually means, you’re not alone. The truth is, not every test will deliver a big win. But that doesn’t mean it’s a wasted effort.
Every result, even an inconclusive one, is a chance to learn. Maybe your hypothesis missed the mark, or maybe the problem lies elsewhere in the journey. That’s why documenting insights and applying a prioritisation framework like PECTI is so important.
Want a clear path forward when results are murky? Our Free A/B Testing Kit includes a simple decision matrix to help you decide when to launch, refine, or retire a test - based on data, not guesswork.
📥 Grab Your Free A/B Testing Kit
FAQs About Shopify A/B Testing
How much do A/B testing platforms cost?
Costs will depend on the platform you choose and the traffic your store receives. Some tools (like Intelligems or Shoplift) charge based on monthly unique visitors, while others (like Convert or VWO) charge only for visitors involved in the actual test.
If you're unsure where to start, platforms like Convert are a solid option for Shopify stores. As a Convert partner, we also have access to a dedicated Partner Dashboard, giving us greater visibility and control when setting up and managing experiments for our clients. Generally, the higher your traffic, the more robust the testing plan you'll need.
How long should an A/B test run?
A/B tests typically need to run for at least two weeks to capture both weekday and weekend behaviours. However, the actual length can vary depending on your traffic levels, sales cycle, and the type of test.
The key is to reach statistical significance, not just a time frame. If your test doesn’t hit significance within two weeks, it may need to run longer. Tools like Convert, VWO, or Intelligems will calculate this for you.
How do I know when my A/B test has enough data?
Before you can confidently determine a winning variation, your test needs a sufficient amount of data. As a general rule, aim for at least 300 conversions per variation. This gives your test a fair chance of producing a statistically valid result.
That said, it's not just about hitting a number. Your A/B testing platform will typically calculate this in real time and show you how close you are to reaching statistical significance.
If you're unsure how to interpret these signals, working with a partner like Blend means we’ll guide you on when to keep running, when to stop, and how to avoid drawing the wrong conclusions too early.
How do I know when I’ve reached statistical significance?
Statistical significance helps you understand whether your A/B test result is reliable or simply a result of chance. Most testing platforms aim for 95% confidence, meaning there's only a 5% likelihood that your result is due to randomness.
You’ll know you’ve reached statistical significance when:
- Your platform identifies a clear winner
- The probability of outperforming the control passes your confidence threshold
- Your results remain consistently strong across segments over time
Keep in mind that significance depends on both sample size and result consistency. Early performance spikes can fade, which is why it's important to let tests run for a minimum of two weeks, even if significance appears early on.
At Blend, we monitor significance daily and provide actionable insights, so you’re not left second-guessing whether your test was truly successful.
Can A/B testing harm my site or user experience?
When implemented properly, A/B testing is completely safe. To mitigate risk:
- Run an AA test first to check for performance or technical issues.
- Start with a small traffic split (e.g. 10%) to monitor stability.
- Avoid testing during major promotions or peak seasons unless necessary.
If you’re working with a partner like Blend, we handle technical QA, rollout, and monitoring to ensure smooth implementation.
Can A/B testing negatively impact my site?
To mitigate risks, we recommend that you first run an AA test to ensure that the A/B testing platform does not introduce technical issues. Additionally, we recommend rolling out tests gradually:
- Start with 10% of traffic to monitor performance.
- Expand to full traffic exposure if no issues arise.
- Monitor throughout to ensure conversion rates and site performance remain stable.
How should I analyse and report on A/B test results?
It’s important to look beyond just the top-line results. Your reporting should include:
- Overall performance: Did the variant improve your key metric?
- Segment performance: How did the test affect mobile vs desktop, or new vs returning visitors?
- Behaviour trends: Was there consistent daily improvement?
- Next steps: Should the variant be launched, retested, or refined?
If you're managing this internally, your A/B testing platform and GA4 will be key tools. At Blend, we provide a full test report including deeper insights and recommendations for future optimisation.
What if my A/B test doesn’t produce a winning result?
Not every A/B test will be a win, and that’s okay. Losing or inconclusive tests still offer valuable insights. They help rule out ineffective ideas, highlight unexpected user behaviour, or guide your next hypothesis.
Rather than seeing it as a wasted effort, consider it a step towards greater clarity. At Blend, we use our PECTI model to reprioritise tests based on what we learn, even from experiments that didn’t go to plan.
Is A/B Testing Worth the Investment
Consider this: if implementing one change increases your conversion rate by 10–20%, what revenue uplift could your business experience annually?
In our work with Pertronix, changing the copy in the product recommendation widget within the Cart Drawer increased the conversion rate by 12.91%, revenue per visitor by 40.37%, and AOV (Average Order Value) by 21.18%. This proves that even small A/B test changes can deliver significant returns.
Strategic A/B testing ensures continuous, measurable improvements, making it an essential investment.
Want to see what's possible with A/B testing?
Book your free CRO strategy call with Blend Commerce today.
Or explore our Shopify CRO Audit or our CRO Program to get started with a data-backed growth strategy.
About the author
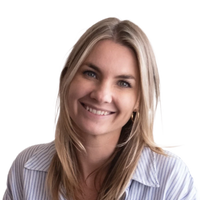
Kelly Managing Director
Our Managing Director, Kelly, is the heart and soul of Blend and embodies warmth and kindness in every aspect of her work. With a remarkable talent for keeping things running like a well-oiled machine, Kelly is the driving force behind our seamless operations. Her commitment to excellence is unwavering, and under her leadership, Blend thrives, and our clients enjoy top-tier service. We’re truly fortunate to have her at the helm, steering us toward success and exceptional customer experiences.
Get useful eCommerce stuff
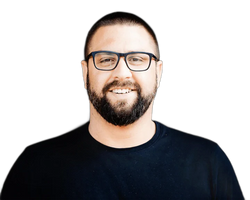